Researchers from the University of Toronto and University of Cambridge are looking into the ways ageism – prejudice against individuals based on age – can be encoded into the technologies and artificial intelligence that many of us use daily.
This age-related bias in AI, also referred to as Digital Ageism, is explored in a new paper led by Charlene Chu, an assistant professor at the Lawrence Bloomberg Faculty of Nursing.
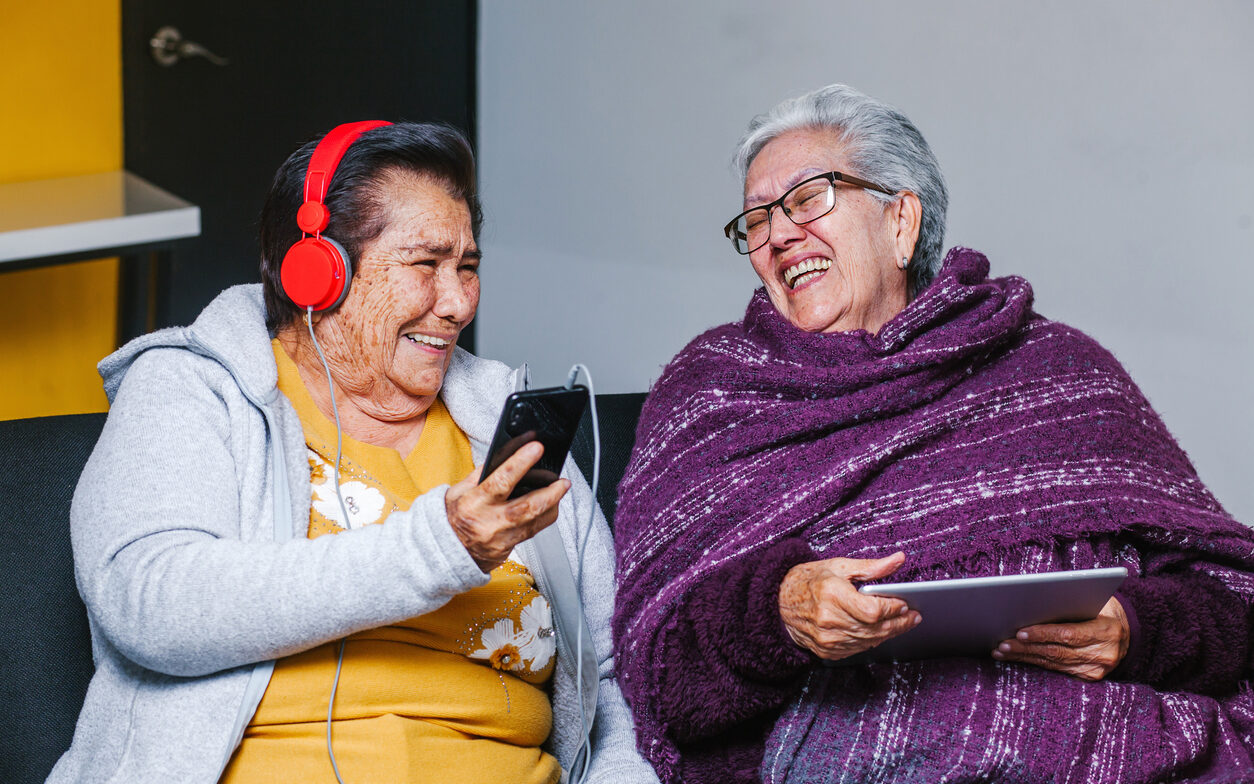
The paper was recently published in the leading journal of gerontology, The Gerontologist.
“The COVID-19 pandemic has heightened our awareness of how dependent our society is on technology. Huge numbers of older adults are turning to technology in their daily lives which has created a sense of urgency for researchers to try to understand digital ageism, and the risks and harms associated with AI biases” says Chu.
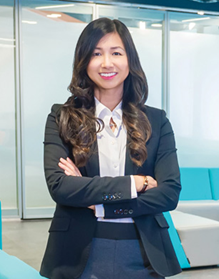
Chu and her research team consisting of legal scholars, computer scientists, philosophers, and social scientists in bioethics and gerontology, note that stereotypes are deeply ingrained in AI algorithms, with recent research focusing on examples of racial and gender-based bias. Solutions to address AI bias, however, are not simple says Chu. She and her team suggest that there are series of “cycles of injustice” that occur in technology development, from early-stage design to testing and implementation.
“Cumulatively, these cycles produce an implicit bias that is baked into the technology’s function which exclude older adults in a disproportionate way,” says Chu.
Read Chu’s discussion on digital ageism in The National Post
Bloomberg Nursing’s Rebecca Biason spoke with Chu about her work and the implications of digital ageism for older adults.
How might technology or apps perpetuate digital ageism?
There are several ways that AI powered technology may take on age related bias, some are more obvious than others. Most apps created for older adults tend to focus on chronic disease and health care management and are rarely associated with pleasure or leisure. Instead, technology created for older adults, tends to view them with a biomedical lens, generating technology that is focused on a health-related need. This ageist representation of older adults trickles into the design of technology, and normal aspects of aging, like differences in motor function or perception, are not taken into consideration. This is one of the “cycles of injustice” that perpetuates age-related bias described in my paper that underpins the exclusion of older adults’ voices and data.
How does the exclusion of older adults contribute to digital ageism?
The data that is used to build various models and algorithms subsequently impacts the performance of the algorithm. Specific to age-related bias, older adults are the fastest growing group of individuals using technology, yet much of the data used to build AI systems are based on younger people. This in turn generates apps and technologies that are not designed for older adults, so they do not use them.
This mismatch in design and technology contributes to a lack of data from older adults, which amplifies their exclusion throughout the pipeline of technology creation.
Ageism is the most socially accepted bias despite that it’s an eventuality for all of us. As the demographic of populations begins to shift, more and more older adults will be turning to technology that is not designed for them. Part of our future work is to effectively illustrate how embedded ageism is within AI and technology development and suggest ways to mitigate that.
What are some of your early recommendations for addressing digital ageism for older adults?
Awareness about digital ageism is the first step and critical to moving forward. Age intersects with other dimensions of vulnerability and should be addressed. A structural recommendation is to discuss the need for interdisciplinary co-design, that is including older adults in technology design from the beginning and not at the end, and data sets that are more representative of older adults.
One thing my team did was comb through The AI Ethics Guidelines Global Inventory which is a repository that compiles recommendation documents about how AI systems can conduct ethical automated decision-making. Many of these guidelines highlighted fairness as a key governing ethical principle, and the need for a reduction of bias. Of these nearly 150 documents created by established organizations, governments, and international groups, we found very little mention of age, age bias, or ageism in comparison to racial or sex related biases.
Now, my team is trying to determine the societal and ethical implications, as well as the degree of harm being done currently with respect to digital ageism. This work is foundational in bringing attention to this issue as we set out to define the problem.